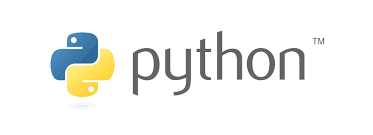
!pip install finance-datareader
!pip install beautifulsoup4
!pip install numpy
!pip install pandas
# default settings
import numpy as np
import pandas as pd
# jupyter notebook 여러 실행인자 실행해도 print되게 만들기
from IPython.core.interactiveshell import InteractiveShell
InteractiveShell.ast_node_interactivity = "all"
pd.set_option('display.float_format', lambda x: '%.3f' % x)
pd.set_option('max_columns', None)
데이터 준비
df = pd.read_csv("data/2016_12.csv")
df.head()
ticker | 매출액(억원) | 영업이익률(%) | 순이익률(%) | 당기순이익(억원) | ROE(%) | ROA(%) | ROIC(%) | EPS(원) | BPS(원) | SPS(원) | PER(배) | PBR(배) | PSR(배) | price | price2 | |
---|---|---|---|---|---|---|---|---|---|---|---|---|---|---|---|---|
0 | AK홀딩스 | 29218.310 | 7.313 | 4.563 | 1333.223 | 12.193 | 5.091 | 15.515 | 5436.413 | 48112.402 | 220556.160 | 10.301 | 1.164 | 0.254 | 56000.000 | 68500.000 |
1 | BGF | 860.773 | 9.315 | 214.481 | 1846.192 | 21.624 | 10.433 | 8.015 | 3703.577 | 18648.623 | 1737.263 | 22.757 | 4.519 | 48.514 | 42140.000 | 15250.000 |
2 | BNK금융지주 | 49126.760 | 14.499 | 10.546 | 5181.144 | 7.919 | 0.564 | NaN | 1568.237 | 20810.660 | 15358.993 | 5.535 | 0.417 | 0.565 | 8680.000 | 9420.000 |
3 | BYC | 2118.576 | 7.625 | 8.281 | 175.433 | 4.543 | 2.463 | 10.748 | 20872.312 | 471887.000 | 252211.450 | 19.020 | 0.841 | 1.574 | 397000.000 | 306000.000 |
4 | CJ | 239541.970 | 5.230 | 2.379 | 5698.234 | 6.080 | 2.253 | 5.158 | 6257.152 | 114276.080 | 672045.900 | 28.181 | 1.543 | 0.262 | 176334.000 | 171148.000 |
# 수익률을 구해보자
# 참고로 price2 : 17.12 종가
# price : 16.12 종가 이다.
df['rtn'] = df['price2'] / df['price'] - 1
df[['ticker', 'rtn']].head()
ticker | rtn | |
---|---|---|
0 | AK홀딩스 | 0.223 |
1 | BGF | -0.638 |
2 | BNK금융지주 | 0.085 |
3 | BYC | -0.229 |
4 | CJ | -0.029 |
값을 기준으로 grouping
# 아래와 같은 네 가지 그룹으로 나눠보자
bound1 = df['PER(배)'] >= 10
bound2 = (5 <= df['PER(배)']) & (df['PER(배)'] < 10)
bound3 = (0 <= df['PER(배)']) & (df['PER(배)'] < 5)
bound4 = df['PER(배)'] < 0
# PER이 10보다 큰 애들만 출력
df[bound1].head()
ticker | 매출액(억원) | 영업이익률(%) | 순이익률(%) | 당기순이익(억원) | ROE(%) | ROA(%) | ROIC(%) | EPS(원) | BPS(원) | SPS(원) | PER(배) | PBR(배) | PSR(배) | price | price2 | rtn | |
---|---|---|---|---|---|---|---|---|---|---|---|---|---|---|---|---|---|
0 | AK홀딩스 | 29218.310 | 7.313 | 4.563 | 1333.223 | 12.193 | 5.091 | 15.515 | 5436.413 | 48112.402 | 220556.160 | 10.301 | 1.164 | 0.254 | 56000.000 | 68500.000 | 0.223 |
1 | BGF | 860.773 | 9.315 | 214.481 | 1846.192 | 21.624 | 10.433 | 8.015 | 3703.577 | 18648.623 | 1737.263 | 22.757 | 4.519 | 48.514 | 42140.000 | 15250.000 | -0.638 |
3 | BYC | 2118.576 | 7.625 | 8.281 | 175.433 | 4.543 | 2.463 | 10.748 | 20872.312 | 471887.000 | 252211.450 | 19.020 | 0.841 | 1.574 | 397000.000 | 306000.000 | -0.229 |
4 | CJ | 239541.970 | 5.230 | 2.379 | 5698.234 | 6.080 | 2.253 | 5.158 | 6257.152 | 114276.080 | 672045.900 | 28.181 | 1.543 | 0.262 | 176334.000 | 171148.000 | -0.029 |
5 | CJ CGV | 14322.454 | 4.911 | 0.393 | 56.217 | 3.105 | 0.284 | 2.919 | 583.051 | 17654.154 | 67682.260 | 120.744 | 3.988 | 1.040 | 70400.000 | 74200.000 | 0.054 |
그룹으로 나눠보자
df.loc[bound1, 'PER_Score'] = 1
df.loc[bound2, 'PER_Score'] = 2
df.loc[bound3, 'PER_Score'] = 3
df.loc[bound4, 'PER_Score'] = -1
df['PER_Score'].head()
0 1.000
1 1.000
2 2.000
3 1.000
4 1.000
Name: PER_Score, dtype: float64
df['PER_Score'].nunique()
4
# 각 그룹별로 몇개인지 출력
df['PER_Score'].value_counts()
1.000 378
2.000 148
-1.000 120
3.000 23
Name: PER_Score, dtype: int64
# nan가 있는지 체크해보자
df['PER_Score'].hasnans
True
# nan이 몇개나 있지?
df['PER_Score'].isna().sum()
12
# PER_Score를 만드는 PER(배)를 먼저 분석해 보자
df['PER(배)'].isna().sum()
12
역시 PER(배)에서 부터 nan이 존재한다
df[df['PER(배)'].isna()]
ticker | 매출액(억원) | 영업이익률(%) | 순이익률(%) | 당기순이익(억원) | ROE(%) | ROA(%) | ROIC(%) | EPS(원) | BPS(원) | SPS(원) | PER(배) | PBR(배) | PSR(배) | price | price2 | rtn | PER_Score | |
---|---|---|---|---|---|---|---|---|---|---|---|---|---|---|---|---|---|---|
27 | HSD엔진 | 8029.166 | 0.528 | -22.571 | -1812.265 | -32.685 | -13.120 | 2.749 | -2607.575 | 7687.264 | 11552.757 | NaN | 0.404 | NaN | 2290.000 | 2569.000 | 0.122 | NaN |
103 | 고려개발 | 6238.648 | 4.223 | -4.895 | -305.362 | 141.454 | -4.903 | 20.462 | -3118.716 | 1988.392 | 63716.438 | NaN | 6.327 | NaN | 12430.000 | 11659.000 | -0.062 | NaN |
126 | 까뮤이앤씨 | 1397.081 | 3.180 | 2.331 | 32.563 | 6.721 | 2.390 | 7.808 | 72.117 | 1153.474 | 3094.153 | NaN | 0.958 | NaN | 1105.000 | 1010.000 | -0.086 | NaN |
133 | 남영비비안 | 2074.953 | -0.949 | -1.103 | -22.895 | -1.667 | -1.371 | -2.101 | -32.324 | 2031.729 | 3021.213 | NaN | 0.436 | NaN | 885.000 | 777.000 | -0.122 | NaN |
203 | 티탑스 | 940.880 | -7.061 | -4.217 | -39.673 | -8.980 | -4.092 | NaN | -1917.146 | 19287.736 | 44614.258 | NaN | 0.058 | NaN | 1115.000 | 1575.000 | 0.413 | NaN |
231 | 롯데칠성음료 | 22642.120 | 6.463 | 3.054 | 691.439 | 2.961 | 1.531 | 3.109 | 5107.448 | 172911.200 | 166829.270 | NaN | 8.363 | NaN | 145675.000 | 132900.000 | -0.088 | NaN |
371 | 쌍용양회공업 | 14302.892 | 18.027 | 12.243 | 1751.111 | 11.589 | 5.694 | 9.037 | 400.919 | 3627.562 | 3319.079 | NaN | 0.816 | NaN | 2960.000 | 3730.000 | 0.260 | NaN |
507 | 컨버즈 | 459.748 | -19.328 | -35.526 | -163.331 | NaN | NaN | NaN | -33811.426 | 20164.525 | 95172.870 | NaN | 0.397 | NaN | 4416.000 | 1750.000 | -0.604 | NaN |
560 | 풀무원 | 20306.730 | 1.869 | 0.491 | 99.778 | 6.765 | 0.979 | 1.933 | 4881.663 | 6986.189 | 533111.700 | NaN | 2.011 | NaN | 14050.000 | 17200.000 | 0.224 | NaN |
622 | 한일철강 | 1112.614 | 5.475 | 4.236 | 47.131 | 3.405 | 1.467 | 4.510 | 249.431 | 8408.528 | 5453.988 | NaN | 0.297 | NaN | 2500.000 | 2645.000 | 0.058 | NaN |
647 | 현대미포조선 | 34464.586 | 5.544 | 1.149 | 395.997 | 1.811 | 0.424 | 21.172 | 865.570 | 53716.750 | 86286.260 | NaN | 0.627 | NaN | 33689.000 | 39454.000 | 0.171 | NaN |
667 | 화천기계 | 1954.730 | -0.135 | -1.282 | -25.052 | -2.012 | -1.559 | -1.413 | -113.874 | 6201.020 | 8885.138 | NaN | 0.348 | NaN | 2160.000 | 2170.000 | 0.005 | NaN |
# PER_Score가 nan일 경우 0으로 채우자
df.loc[df['PER_Score'].isna(), "PER_Score"] = 0
# 혹은 이렇게 가능
# df['PER_Score'] = df['PER_Score'].fillna(0)
df.loc[:, "PER_Score1"] = (bound1 * 1) + (bound2 * 2) + (bound3 * 3) + (bound4 * -1)
df['PER_Score1'].head()
0 1
1 1
2 2
3 1
4 1
Name: PER_Score1, dtype: int32
# 이런식으로 처리시 nan이 애초에 존재하지 않는다
df['PER_Score1'].hasnans
False
# 두 PER Score는 같을까?
df['PER_Score'].equals(df['PER_Score1'])
False
# 데이터 타입자체가 다르다
df['PER_Score'].dtypes
df['PER_Score1'].dtypes
dtype('float64')
dtype('int32')
# 데이터형을 통일시키면 같음.
df['PER_Score'].astype(int).equals(df['PER_Score1'])
True
구간 나누기 함수 cut()
per_cuts = pd.cut(
df['PER(배)'],
[-np.inf, 0, 5, 10, np.inf],
# -np.inf, 0 / 0, 5 / 5, 10 / 10, np.inf
# 구간 중 하나로
)
per_cuts.head()
0 (10.0, inf]
1 (10.0, inf]
2 (5.0, 10.0]
3 (10.0, inf]
4 (10.0, inf]
Name: PER(배), dtype: category
Categories (4, interval[float64, right]): [(-inf, 0.0] < (0.0, 5.0] < (5.0, 10.0] < (10.0, inf]]
# Interval이라는 자료형으로 들어가게 됨.
per_cuts.iloc[0]
Interval(10.0, inf, closed='right')
# 각 몇개씩 들어가 있는지 보자면.
per_cuts.value_counts()
(10.0, inf] 378
(5.0, 10.0] 148
(-inf, 0.0] 120
(0.0, 5.0] 23
Name: PER(배), dtype: int64
per_cuts.isna().sum()
12
bins = [-np.inf, 10, 20, np.inf]
labels = ['저평가주', '보통주', '고평가주']
per_cuts2 = pd.cut(
df['PER(배)'],
bins=bins,
labels=labels
)
per_cuts2.head()
0 보통주
1 고평가주
2 저평가주
3 보통주
4 고평가주
Name: PER(배), dtype: category
Categories (3, object): ['저평가주' < '보통주' < '고평가주']
개체수 기준으로 grouping - qcut()
# qcut
pd.qcut(df['PER(배)'], 3, labels=[1,2,3]).head()
# pd.qcut(df['PER(배)'], 3, labels=[1,2,3]).value_counts()
0 2
1 3
2 1
3 3
4 3
Name: PER(배), dtype: category
Categories (3, int64): [1 < 2 < 3]
df.loc[:, 'PER_Score2'] = pd.qcut(df['PER(배)'], 10, labels=range(1, 11))
df.head()
ticker | 매출액(억원) | 영업이익률(%) | 순이익률(%) | 당기순이익(억원) | ROE(%) | ROA(%) | ROIC(%) | EPS(원) | BPS(원) | SPS(원) | PER(배) | PBR(배) | PSR(배) | price | price2 | rtn | PER_Score | PER_Score1 | PER_Score2 | |
---|---|---|---|---|---|---|---|---|---|---|---|---|---|---|---|---|---|---|---|---|
0 | AK홀딩스 | 29218.310 | 7.313 | 4.563 | 1333.223 | 12.193 | 5.091 | 15.515 | 5436.413 | 48112.402 | 220556.160 | 10.301 | 1.164 | 0.254 | 56000.000 | 68500.000 | 0.223 | 1.000 | 1 | 5 |
1 | BGF | 860.773 | 9.315 | 214.481 | 1846.192 | 21.624 | 10.433 | 8.015 | 3703.577 | 18648.623 | 1737.263 | 22.757 | 4.519 | 48.514 | 42140.000 | 15250.000 | -0.638 | 1.000 | 1 | 8 |
2 | BNK금융지주 | 49126.760 | 14.499 | 10.546 | 5181.144 | 7.919 | 0.564 | NaN | 1568.237 | 20810.660 | 15358.993 | 5.535 | 0.417 | 0.565 | 8680.000 | 9420.000 | 0.085 | 2.000 | 2 | 3 |
3 | BYC | 2118.576 | 7.625 | 8.281 | 175.433 | 4.543 | 2.463 | 10.748 | 20872.312 | 471887.000 | 252211.450 | 19.020 | 0.841 | 1.574 | 397000.000 | 306000.000 | -0.229 | 1.000 | 1 | 8 |
4 | CJ | 239541.970 | 5.230 | 2.379 | 5698.234 | 6.080 | 2.253 | 5.158 | 6257.152 | 114276.080 | 672045.900 | 28.181 | 1.543 | 0.262 | 176334.000 | 171148.000 | -0.029 | 1.000 | 1 | 9 |
df['PER_Score2'].value_counts()
1 67
2 67
3 67
4 67
5 67
7 67
8 67
9 67
10 67
6 66
Name: PER_Score2, dtype: int64
# 역시 nan는 있다
df['PER_Score2'].hasnans
True
df = df.dropna(subset=['PER(배)'])
df['PER_Score2'].isna().sum()
0
데이터 세팅
df = pd.read_csv("data/2016_12.csv")
df = df.dropna()
g_df = df.copy()
g_df['rtn'] = g_df['price2'] / g_df['price'] - 1
g_df.head()
ticker | 매출액(억원) | 영업이익률(%) | 순이익률(%) | 당기순이익(억원) | ROE(%) | ROA(%) | ROIC(%) | EPS(원) | BPS(원) | SPS(원) | PER(배) | PBR(배) | PSR(배) | price | price2 | rtn | |
---|---|---|---|---|---|---|---|---|---|---|---|---|---|---|---|---|---|
0 | AK홀딩스 | 29218.310 | 7.313 | 4.563 | 1333.223 | 12.193 | 5.091 | 15.515 | 5436.413 | 48112.402 | 220556.160 | 10.301 | 1.164 | 0.254 | 56000.000 | 68500.000 | 0.223 |
1 | BGF | 860.773 | 9.315 | 214.481 | 1846.192 | 21.624 | 10.433 | 8.015 | 3703.577 | 18648.623 | 1737.263 | 22.757 | 4.519 | 48.514 | 42140.000 | 15250.000 | -0.638 |
3 | BYC | 2118.576 | 7.625 | 8.281 | 175.433 | 4.543 | 2.463 | 10.748 | 20872.312 | 471887.000 | 252211.450 | 19.020 | 0.841 | 1.574 | 397000.000 | 306000.000 | -0.229 |
4 | CJ | 239541.970 | 5.230 | 2.379 | 5698.234 | 6.080 | 2.253 | 5.158 | 6257.152 | 114276.080 | 672045.900 | 28.181 | 1.543 | 0.262 | 176334.000 | 171148.000 | -0.029 |
5 | CJ CGV | 14322.454 | 4.911 | 0.393 | 56.217 | 3.105 | 0.284 | 2.919 | 583.051 | 17654.154 | 67682.260 | 120.744 | 3.988 | 1.040 | 70400.000 | 74200.000 | 0.054 |
g_df.loc[:, 'PER_score'] = pd.qcut(g_df['PER(배)'], 10, labels=range(1, 11))
g_df.loc[:, 'PBR_score'] = pd.qcut(g_df['PBR(배)'], 10, labels=range(1, 11))
g_df.set_index('ticker', inplace=True)
g_df.head()
매출액(억원) | 영업이익률(%) | 순이익률(%) | 당기순이익(억원) | ROE(%) | ROA(%) | ROIC(%) | EPS(원) | BPS(원) | SPS(원) | PER(배) | PBR(배) | PSR(배) | price | price2 | rtn | PER_score | PBR_score | |
---|---|---|---|---|---|---|---|---|---|---|---|---|---|---|---|---|---|---|
ticker | ||||||||||||||||||
AK홀딩스 | 29218.310 | 7.313 | 4.563 | 1333.223 | 12.193 | 5.091 | 15.515 | 5436.413 | 48112.402 | 220556.160 | 10.301 | 1.164 | 0.254 | 56000.000 | 68500.000 | 0.223 | 5 | 7 |
BGF | 860.773 | 9.315 | 214.481 | 1846.192 | 21.624 | 10.433 | 8.015 | 3703.577 | 18648.623 | 1737.263 | 22.757 | 4.519 | 48.514 | 42140.000 | 15250.000 | -0.638 | 8 | 10 |
BYC | 2118.576 | 7.625 | 8.281 | 175.433 | 4.543 | 2.463 | 10.748 | 20872.312 | 471887.000 | 252211.450 | 19.020 | 0.841 | 1.574 | 397000.000 | 306000.000 | -0.229 | 8 | 5 |
CJ | 239541.970 | 5.230 | 2.379 | 5698.234 | 6.080 | 2.253 | 5.158 | 6257.152 | 114276.080 | 672045.900 | 28.181 | 1.543 | 0.262 | 176334.000 | 171148.000 | -0.029 | 9 | 8 |
CJ CGV | 14322.454 | 4.911 | 0.393 | 56.217 | 3.105 | 0.284 | 2.919 | 583.051 | 17654.154 | 67682.260 | 120.744 | 3.988 | 1.040 | 70400.000 | 74200.000 | 0.054 | 10 | 10 |
groupby() vs aggregation()
groupby()
# "PBR_score", "PER_score"로 group을 생성해 달라
# 예를들어 PBR_score(1), PER_score(2) 인 그룹
# PBR_score(1), PER_score(3) 인 그룹
# ...
g_df_obj = g_df.groupby(["PBR_score", "PER_score"])
g_df_obj
<pandas.core.groupby.generic.DataFrameGroupBy object at 0x000001A52D250EB0>
# 타입조사
type(g_df_obj)
pandas.core.groupby.generic.DataFrameGroupBy
# 몇개의 그룹이 나올까?
g_df_obj.ngroups
96
g_df['PBR_score'].nunique()
g_df['PER_score'].nunique()
10
10
# 몇개씩 들어가 있는지 확인
g_df_obj.size().head()
PBR_score PER_score
1 1 5
2 11
3 11
4 11
5 7
dtype: int64
# Multi-level index를 가진 Series indexing하는 법
g_df_obj.size().loc[1]
g_df_obj.size().loc[2]
g_df_obj.size().loc[(1, 1)]
PER_score
1 5
2 11
3 11
4 11
5 7
6 2
7 4
8 0
9 3
10 7
dtype: int64
PER_score
1 6
2 2
3 10
4 9
5 8
6 7
7 5
8 5
9 5
10 4
dtype: int64
5
# 좀 보기 편하게 하기 위해서
# Series -> DataFrame으로 변환
g_df_obj.size().to_frame().head()
0 | ||
---|---|---|
PBR_score | PER_score | |
1 | 1 | 5 |
2 | 11 | |
3 | 11 | |
4 | 11 | |
5 | 7 |
# 이런식으로 keys와 value를 볼 수 있는데 너무 길어서 생략.
# g_df_obj.groups.keys()
# g_df_obj.groups.values()
# 그룹에 속한 아이템 확인가능.
g_df_obj.get_group((1, 1))
매출액(억원) | 영업이익률(%) | 순이익률(%) | 당기순이익(억원) | ROE(%) | ROA(%) | ROIC(%) | EPS(원) | BPS(원) | SPS(원) | PER(배) | PBR(배) | PSR(배) | price | price2 | rtn | PER_score | PBR_score | |
---|---|---|---|---|---|---|---|---|---|---|---|---|---|---|---|---|---|---|
ticker | ||||||||||||||||||
E1 | 39959.008 | 0.277 | -0.949 | -379.397 | -2.350 | -1.076 | -1.633 | -3869.011 | 191789.690 | 582492.800 | -16.206 | 0.327 | 0.108 | 62700.000 | 56900.000 | -0.093 | 1 | 1 |
S&T중공업 | 4680.078 | -0.309 | -5.221 | -244.358 | -3.671 | -2.697 | -0.791 | -734.851 | 21043.785 | 14074.280 | -12.369 | 0.432 | 0.646 | 9089.000 | 7310.000 | -0.196 | 1 | 1 |
디아이동일 | 8224.145 | 2.100 | 0.132 | 10.885 | -0.753 | 0.111 | 0.051 | -1523.407 | 270773.030 | 333554.280 | -37.365 | 0.210 | 0.171 | 56922.000 | 51884.000 | -0.089 | 1 | 1 |
한국수출포장공업 | 2282.604 | 1.344 | -0.091 | -2.075 | -0.092 | -0.069 | 0.152 | -51.880 | 56026.280 | 57065.100 | -345.030 | 0.319 | 0.314 | 17900.000 | 15650.000 | -0.126 | 1 | 1 |
휴스틸 | 3640.215 | 0.370 | -0.153 | -5.562 | -0.143 | -0.101 | 0.059 | -80.399 | 55779.490 | 52614.773 | -191.546 | 0.276 | 0.293 | 15400.000 | 15450.000 | 0.003 | 1 | 1 |
# for문을 통해 출력
for name, group in g_df_obj:
name
group.head()
break
(1, 1)
매출액(억원) | 영업이익률(%) | 순이익률(%) | 당기순이익(억원) | ROE(%) | ROA(%) | ROIC(%) | EPS(원) | BPS(원) | SPS(원) | PER(배) | PBR(배) | PSR(배) | price | price2 | rtn | PER_score | PBR_score | |
---|---|---|---|---|---|---|---|---|---|---|---|---|---|---|---|---|---|---|
ticker | ||||||||||||||||||
E1 | 39959.008 | 0.277 | -0.949 | -379.397 | -2.350 | -1.076 | -1.633 | -3869.011 | 191789.690 | 582492.800 | -16.206 | 0.327 | 0.108 | 62700.000 | 56900.000 | -0.093 | 1 | 1 |
S&T중공업 | 4680.078 | -0.309 | -5.221 | -244.358 | -3.671 | -2.697 | -0.791 | -734.851 | 21043.785 | 14074.280 | -12.369 | 0.432 | 0.646 | 9089.000 | 7310.000 | -0.196 | 1 | 1 |
디아이동일 | 8224.145 | 2.100 | 0.132 | 10.885 | -0.753 | 0.111 | 0.051 | -1523.407 | 270773.030 | 333554.280 | -37.365 | 0.210 | 0.171 | 56922.000 | 51884.000 | -0.089 | 1 | 1 |
한국수출포장공업 | 2282.604 | 1.344 | -0.091 | -2.075 | -0.092 | -0.069 | 0.152 | -51.880 | 56026.280 | 57065.100 | -345.030 | 0.319 | 0.314 | 17900.000 | 15650.000 | -0.126 | 1 | 1 |
휴스틸 | 3640.215 | 0.370 | -0.153 | -5.562 | -0.143 | -0.101 | 0.059 | -80.399 | 55779.490 | 52614.773 | -191.546 | 0.276 | 0.293 | 15400.000 | 15450.000 | 0.003 | 1 | 1 |
g_df.groupby('PER_score').size()
g_df.groupby('PER_score').head(1).head(5)
PER_score
1 61
2 61
3 61
4 61
5 61
6 60
7 61
8 61
9 61
10 61
dtype: int64
매출액(억원) | 영업이익률(%) | 순이익률(%) | 당기순이익(억원) | ROE(%) | ROA(%) | ROIC(%) | EPS(원) | BPS(원) | SPS(원) | PER(배) | PBR(배) | PSR(배) | price | price2 | rtn | PER_score | PBR_score | |
---|---|---|---|---|---|---|---|---|---|---|---|---|---|---|---|---|---|---|
ticker | ||||||||||||||||||
AK홀딩스 | 29218.310 | 7.313 | 4.563 | 1333.223 | 12.193 | 5.091 | 15.515 | 5436.413 | 48112.402 | 220556.160 | 10.301 | 1.164 | 0.254 | 56000.000 | 68500.000 | 0.223 | 5 | 7 |
BGF | 860.773 | 9.315 | 214.481 | 1846.192 | 21.624 | 10.433 | 8.015 | 3703.577 | 18648.623 | 1737.263 | 22.757 | 4.519 | 48.514 | 42140.000 | 15250.000 | -0.638 | 8 | 10 |
CJ | 239541.970 | 5.230 | 2.379 | 5698.234 | 6.080 | 2.253 | 5.158 | 6257.152 | 114276.080 | 672045.900 | 28.181 | 1.543 | 0.262 | 176334.000 | 171148.000 | -0.029 | 9 | 8 |
CJ CGV | 14322.454 | 4.911 | 0.393 | 56.217 | 3.105 | 0.284 | 2.919 | 583.051 | 17654.154 | 67682.260 | 120.744 | 3.988 | 1.040 | 70400.000 | 74200.000 | 0.054 | 10 | 10 |
DB | 2048.100 | 5.324 | -10.113 | -207.128 | -13.052 | -8.434 | 66.574 | -113.728 | 881.178 | 1124.549 | -6.454 | 0.833 | 0.653 | 734.000 | 658.000 | -0.104 | 2 | 5 |
aggreggation()
g_df.groupby("PBR_score").agg(
{
"rtn": "mean", # = np.mean
}
)
rtn | |
---|---|
PBR_score | |
1 | -0.001 |
2 | 0.020 |
3 | -0.021 |
4 | 0.161 |
5 | -0.012 |
6 | -0.043 |
7 | 0.150 |
8 | 0.058 |
9 | 0.139 |
10 | 0.054 |
pbr_rtn_df = g_df.groupby("PBR_score").agg({'rtn': 'mean'})
per_rtn_df = g_df.groupby("PER_score").agg({'rtn': 'mean'})
pbr_rtn_df.head()
rtn | |
---|---|
PBR_score | |
1 | -0.001 |
2 | 0.020 |
3 | -0.021 |
4 | 0.161 |
5 | -0.012 |
# 다양한 방법으로 진행하기 (같은 결과)
g_df.groupby("PER_score")['rtn'].agg('mean').head()
g_df.groupby("PER_score")['rtn'].agg(np.mean).head()
g_df.groupby("PER_score")['rtn'].mean().head()
PER_score
1 -0.062
2 -0.083
3 -0.038
4 0.056
5 0.000
Name: rtn, dtype: float64
PER_score
1 -0.062
2 -0.083
3 -0.038
4 0.056
5 0.000
Name: rtn, dtype: float64
PER_score
1 -0.062
2 -0.083
3 -0.038
4 0.056
5 0.000
Name: rtn, dtype: float64
# 단, return type이 다를 수 있음에 주의
g_df.groupby("PER_score")['rtn'].agg("mean").head(2) # Series로 return
g_df.groupby("PER_score")[['rtn']].agg("mean").head(2) # DataFrame으로 return
PER_score
1 -0.062
2 -0.083
Name: rtn, dtype: float64
rtn | |
---|---|
PER_score | |
1 | -0.062 |
2 | -0.083 |
# 2개 이상의 컬럼에 대해 aggregation
g_df.groupby("PER_score")[['rtn', 'PBR(배)']].agg("mean").head(2)
rtn | PBR(배) | |
---|---|---|
PER_score | ||
1 | -0.062 | 1.839 |
2 | -0.083 | 1.323 |
# 2개 이상의 aggregation
g_df.groupby("PER_score")[['rtn', 'PBR(배)']].agg(["mean", "std"]).head(2)
rtn | PBR(배) | |||
---|---|---|---|---|
mean | std | mean | std | |
PER_score | ||||
1 | -0.062 | 0.328 | 1.839 | 2.215 |
2 | -0.083 | 0.781 | 1.323 | 1.167 |
# 2개 이상의 컬럼 & 각각에 대해 다른 aggregation
g_df.groupby("PBR_score").agg(
{
'rtn': ['mean', 'std'],
'PER(배)': ['min']
}
)
rtn | PER(배) | ||
---|---|---|---|
mean | std | min | |
PBR_score | |||
1 | -0.001 | 0.262 | -345.030 |
2 | 0.020 | 0.280 | -319.356 |
3 | -0.021 | 0.219 | -10.614 |
4 | 0.161 | 0.770 | -938.983 |
5 | -0.012 | 0.265 | -62.397 |
6 | -0.043 | 0.251 | -310.606 |
7 | 0.150 | 0.704 | -107.741 |
8 | 0.058 | 0.472 | -27857.496 |
9 | 0.139 | 0.669 | -352.735 |
10 | 0.054 | 0.464 | -442.464 |
# sqrt는 aggregation 방식의 연산이 아님!
np.sqrt([1, 2, 3, 4])
array([1. , 1.41421356, 1.73205081, 2. ])
# g_df.groupby("PER_score")['rtn'].agg(np.sqrt)
# error
!pip install matplotlib
pbr_rtn_df.plot(kind='bar')
Collecting matplotlib
Using cached matplotlib-3.5.1-cp310-cp310-win_amd64.whl (7.2 MB)
Requirement already satisfied: pyparsing>=2.2.1 in c:\git\python-data\venv\lib\site-packages (from matplotlib) (3.0.6)
Collecting kiwisolver>=1.0.1
Using cached kiwisolver-1.3.2-cp310-cp310-win_amd64.whl (52 kB)
Collecting pillow>=6.2.0
Using cached Pillow-8.4.0-cp310-cp310-win_amd64.whl (3.2 MB)
Requirement already satisfied: python-dateutil>=2.7 in c:\git\python-data\venv\lib\site-packages (from matplotlib) (2.8.2)
Requirement already satisfied: numpy>=1.17 in c:\git\python-data\venv\lib\site-packages (from matplotlib) (1.21.5)
Collecting fonttools>=4.22.0
Using cached fonttools-4.28.5-py3-none-any.whl (890 kB)
Requirement already satisfied: packaging>=20.0 in c:\git\python-data\venv\lib\site-packages (from matplotlib) (21.3)
Collecting cycler>=0.10
Using cached cycler-0.11.0-py3-none-any.whl (6.4 kB)
Requirement already satisfied: six>=1.5 in c:\git\python-data\venv\lib\site-packages (from python-dateutil>=2.7->matplotlib) (1.16.0)
Installing collected packages: pillow, kiwisolver, fonttools, cycler, matplotlib
Successfully installed cycler-0.11.0 fonttools-4.28.5 kiwisolver-1.3.2 matplotlib-3.5.1 pillow-8.4.0
<AxesSubplot:xlabel='PBR_score'>
pbr_rtn_df.plot(kind='bar');
per_rtn_df.plot(kind='bar');
Example
g_df1 = g_df.groupby(["PBR_score", "PER_score"])\
.agg(
{
'rtn': ['mean', 'std', 'min', 'max'],
'ROE(%)': [np.mean, 'size', 'nunique', 'idxmax']
}
)
g_df1.head()
rtn | ROE(%) | ||||||||
---|---|---|---|---|---|---|---|---|---|
mean | std | min | max | mean | size | nunique | idxmax | ||
PBR_score | PER_score | ||||||||
1 | 1 | -0.100 | 0.072 | -0.196 | 0.003 | -1.402 | 5 | 5 | 한국수출포장공업 |
2 | -0.093 | 0.266 | -0.482 | 0.437 | 154.967 | 11 | 11 | 삼부토건 | |
3 | 0.117 | 0.359 | -0.556 | 0.683 | 6.952 | 11 | 11 | 한국전력공사 | |
4 | 0.106 | 0.295 | -0.273 | 0.882 | 5.104 | 11 | 11 | 한국공항 | |
5 | -0.039 | 0.120 | -0.206 | 0.113 | 3.941 | 7 | 7 | 성창기업지주 |
a = g_df.groupby(["PBR_score", "PER_score"])['rtn', 'ROE(%)'].agg(['sum', 'mean'])
C:\Users\TAEHYU~1\AppData\Local\Temp/ipykernel_13144/3376508614.py:1: FutureWarning: Indexing with multiple keys (implicitly converted to a tuple of keys) will be deprecated, use a list instead.
a = g_df.groupby(["PBR_score", "PER_score"])['rtn', 'ROE(%)'].agg(['sum', 'mean'])
# Multi-index라고 해서 쫄 것 없음!
a.loc[1]
a.loc[(1, 3)]
a.loc[[(1, 3), (1, 4 )]]
rtn | ROE(%) | |||
---|---|---|---|---|
sum | mean | sum | mean | |
PER_score | ||||
1 | -0.499 | -0.100 | -7.009 | -1.402 |
2 | -1.025 | -0.093 | 1704.634 | 154.967 |
3 | 1.290 | 0.117 | 76.477 | 6.952 |
4 | 1.165 | 0.106 | 56.146 | 5.104 |
5 | -0.275 | -0.039 | 27.590 | 3.941 |
6 | -0.666 | -0.333 | 6.710 | 3.355 |
7 | -0.126 | -0.031 | 11.435 | 2.859 |
8 | 0.000 | NaN | 0.000 | NaN |
9 | -0.175 | -0.058 | 3.039 | 1.013 |
10 | 0.226 | 0.032 | 2.873 | 0.410 |
rtn sum 1.290
mean 0.117
ROE(%) sum 76.477
mean 6.952
Name: (1, 3), dtype: float64
rtn | ROE(%) | ||||
---|---|---|---|---|---|
sum | mean | sum | mean | ||
PBR_score | PER_score | ||||
1 | 3 | 1.290 | 0.117 | 76.477 | 6.952 |
4 | 1.165 | 0.106 | 56.146 | 5.104 |
- 주의: nan은 groupby시 자동으로 filter out 되기 때문에, 미리 전처리 다 하는게 좋음
df = pd.DataFrame({
'a':['소형주', np.nan, '대형주', '대형주'],
'b':[np.nan, 2, 3, np.nan],
})
df
a | b | |
---|---|---|
0 | 소형주 | NaN |
1 | NaN | 2.000 |
2 | 대형주 | 3.000 |
3 | 대형주 | NaN |
df.groupby(['a'])['b'].mean()
a
대형주 3.000
소형주 NaN
Name: b, dtype: float64
as_index = False
: group cols들이 index가 아니라 하나의 col이 됨 (aggregate하고 reset_index()를 취한 것)
a = g_df.groupby(["PER_score"] ).agg({'rtn': ['mean', 'std']}).head(2)
b = g_df.groupby(["PER_score"], as_index=False).agg({'rtn': ['mean', 'std']}).head(2)
a
b
rtn | ||
---|---|---|
mean | std | |
PER_score | ||
1 | -0.062 | 0.328 |
2 | -0.083 | 0.781 |
PER_score | rtn | ||
---|---|---|---|
mean | std | ||
0 | 1 | -0.062 | 0.328 |
1 | 2 | -0.083 | 0.781 |
a['rtn']
a[('rtn', 'mean')].head()
mean | std | |
---|---|---|
PER_score | ||
1 | -0.062 | 0.328 |
2 | -0.083 | 0.781 |
PER_score
1 -0.062
2 -0.083
Name: (rtn, mean), dtype: float64
Example2
a_df = pd.read_csv("data/Small_and_Big.csv", index_col=[0])
a_df.head()
date | 종목명 | PBR(IFRS-연결) | 베타 (M,5Yr) | 수익률(%) | 시가총액 (보통)(평균)(원) | |
---|---|---|---|---|---|---|
0 | 2000-07-31 | BYC | 0.210 | 0.479 | -0.580 | 27786000000.000 |
1 | 2000-07-31 | CJ | 0.510 | 1.166 | -9.000 | 1160889000000.000 |
2 | 2000-07-31 | CJ ENM | 6.560 | NaN | 17.400 | 400467000000.000 |
3 | 2000-07-31 | CJ대한통운 | 0.170 | 1.314 | -7.960 | 194962000000.000 |
4 | 2000-07-31 | CJ씨푸드 | NaN | 0.227 | 32.000 | 1987000000.000 |
median_df = a_df.groupby(['date']).agg({'시가총액 (보통)(평균)(원)': 'median'})
median_df.head()
시가총액 (보통)(평균)(원) | |
---|---|
date | |
2000-07-31 | 34947000000.000 |
2000-08-31 | 33684000000.000 |
2000-09-30 | 33684000000.000 |
2000-10-31 | 30523000000.000 |
2000-11-30 | 30798000000.000 |
median_df.columns = ['시가총액_median']
median_df.head()
시가총액_median | |
---|---|
date | |
2000-07-31 | 34947000000.000 |
2000-08-31 | 33684000000.000 |
2000-09-30 | 33684000000.000 |
2000-10-31 | 30523000000.000 |
2000-11-30 | 30798000000.000 |